[1]:
%matplotlib inline
OpenML Datasets: Plant Shapes Example¶
How to list and download datasets.
[2]:
import openml
import pandas as pd
from rerf.rerfClassifier import rerfClassifier
# Import scikit-learn dataset library
from sklearn import datasets
# Import train_test_split function
from sklearn.model_selection import train_test_split
RS = 21208
Download datasets¶
[3]:
# This is done based on the dataset ID ('did').
dataset = openml.datasets.get_dataset(1492)
# Print a summary
print("This is dataset '%s', the target feature is '%s'" %
(dataset.name, dataset.default_target_attribute))
print("URL: %s" % dataset.url)
print(dataset.description[:500])
This is dataset 'one-hundred-plants-shape', the target feature is 'Class'
URL: https://www.openml.org/data/v1/download/1592284/one-hundred-plants-shape.arff
**Author**: James Cope, Thibaut Beghin, Paolo Remagnino, Sarah Barman.
**Source**: [UCI](https://archive.ics.uci.edu/ml/datasets/One-hundred+plant+species+leaves+data+set) - 2010
**Please cite**: Charles Mallah, James Cope, James Orwell. Plant Leaf Classification Using Probabilistic Integration of Shape, Texture and Margin Features. Signal Processing, Pattern Recognition and Applications, in press. 2013.
### Description
One-hundred plant species leaves dataset (Class = Shape).
###
Get the actual data.
Returned as numpy array, with meta-info (e.g. target feature, feature names,…)
[4]:
X, y, attribute_names,_ = dataset.get_data(
target=dataset.default_target_attribute
)
dat = pd.DataFrame(X)
Y = [int(yi) - 1 for yi in y]
# Split dataset into training set and test set
X_train, X_test, y_train, y_test = train_test_split(
X, Y, test_size=0.25, random_state = RS
) # 75% training and 25% test
- Explore the data visually.
[5]:
dat = dat.sample(n=1000, random_state = RS)
_ = pd.plotting.scatter_matrix(
dat.iloc[:100, :8],
c=dat[:100]['V64'],
figsize=(10, 10),
marker='o',
hist_kwds={'bins': 20},
alpha=.8,
cmap='magma'
)
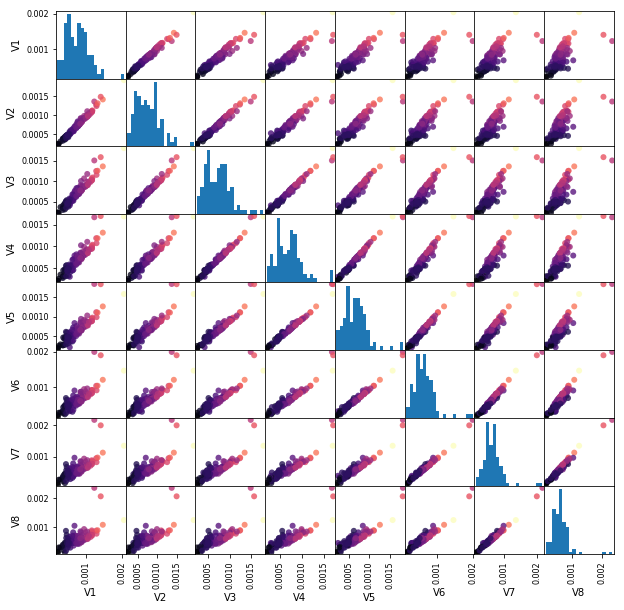
[6]:
# Create a RerF Classifier
clf = rerfClassifier(n_estimators=500, max_features=16, feature_combinations=4, n_jobs = 2, random_state = RS)
[7]:
clf.fit(X_train, y_train)
[7]:
rerfClassifier(feature_combinations=4, image_height=None, image_width=None,
max_depth=None, max_features=16, min_samples_split=1,
n_estimators=500, n_jobs=2, oob_score=False,
patch_height_max=None, patch_height_min=1, patch_width_max=None,
patch_width_min=1, projection_matrix='RerF', random_state=21208)
[8]:
y_pred = clf.predict(X_test)
[9]:
# Import scikit-learn metrics module for accuracy calculation
from sklearn import metrics
[10]:
# Model Accuracy, how often is the classifier correct?
print("Accuracy:", metrics.accuracy_score(y_test, y_pred))
Accuracy: 0.6525